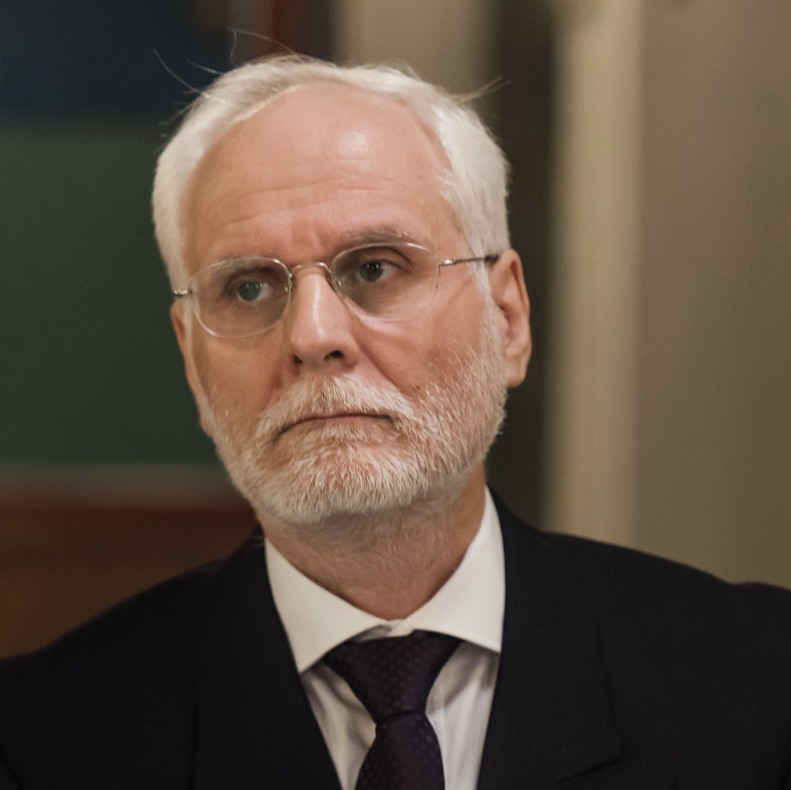
Professor (Lab Director)
George Vouros
Faculty Member
Research Interests
Knowledge Representation & Reasoning with Ontologies, Semantic Enrichment and Transformation of Data, Multiagent Systems, Collaborative Multiagent Reinforcement Learning, Imitation learning
Contact
georgev(-at-)unipi(-dot-)gr
Additional Information
George Vouros is Professor at the Department of Digital Systems, ICT School, University of Piraeus; head of the Artificial Intelligence Laboratory (http://ai-group.ds.unipi.gr).
He holds BSc in Mathemetics and Ph.D. in Computer Science (Artificial Intelligence), both from the National Kapodistrian University of Athens, Greece.
His research interests include Knowledge Representation and Reasoning (ontologies, ontology engineering, scalable alignment, modularization and reasoning with large ontologies), Agents and Multiagent Systems, Reinforcement Learning, Imitation Learning and Human-Centric AI .
He teaches Agents & Multiagent Systems and Artificial Intelligence at pre-graduate level, and Agents & Multiagent Systems, Reinforcement Learning at post-graduate level.
He is or was principal investigator / senior researcher for a number of EU-funded and National research projects (GSRT/AMINESS, FP7/Grid4All, FP7/SEMAGROW, FP7/NOMAD, COST/Agreement Technologies, datACRON on Big Data (ICT-16), DART on data-driven trajectory predictions, TAPAS and SIMBAD).
He has served as co-chair of Agreement Technologies, ESAW, EUMAS, IAT/WI, SETN, COIN conferences and workshops and he served/serves as member of steering committee of workshop and conference series, program committee member for numerous conferences, including ECAI, IJCAI, AAAI, CIKM, AAMAS, WWW, Web Intelligence, SETN.
Among the most recent academic activities he is cochair of SETN 2024, co-organizes ISWC 2023, and will serve the organizing committee of ECAI 2027.
He serves as reviewer in prominent journals for data and knowledge engineering, semantic web and multiagent systems. He has delivered invited lectures and seminars on the topic of computing semantic agreements and multi agent systems. He has co-edited post-proceedings and special issues in topics of his research interests and have published more than 150 refereed articles in scientific journals and conferences.
He currently (2023-2024) serves and has served as president of the Hellenic Artificial Intelligence Society (EETN) from 2006-2010 (four years- two consecutive periods) and 2015-2016.
He is the founder and director of the MSc on AI, co-organized by University of Piraeus and NCSR Demokritos.
Selected Publications
2023 |
Vouros, George A Explainable Deep Reinforcement Learning: State of the Art and Challenges Journal Article ACM Computing Surveys, 55 (5), pp. 1-39, 2023. @article{Vouros2023, title = {Explainable Deep Reinforcement Learning: State of the Art and Challenges}, author = {George A. Vouros}, doi = {10.1145/3527448}, year = {2023}, date = {2023-01-01}, journal = {ACM Computing Surveys}, volume = {55}, number = {5}, pages = {1-39}, abstract = {Interpretability, explainability, and transparency are key issues to introducing artificial intelligence methods in many critical domains. This is important due to ethical concerns and trust issues strongly connected to reliability, robustness, auditability, and fairness, and has important consequences toward keeping the human in the loop in high levels of automation, especially in critical cases for decision making, where both (human and the machine) play important roles. Although the research community has given much attention to explainability of closed (or black) prediction boxes, there are tremendous needs for explainability of closed-box methods that support agents to act autonomously in the real world. Reinforcement learning methods, and especially their deep versions, are such closed-box methods. In this article, we aim to provide a review of state-of-the-art methods for explainable deep reinforcement learning methods, taking also into account the needs of human operators—that is, of those who make the actual and critical decisions in solving real-world problems. We provide a formal specification of the deep reinforcement learning explainability problems, and we identify the necessary components of a general explainable reinforcement learning framework. Based on these, we provide a comprehensive review of state-of-the-art methods, categorizing them into classes according to the paradigm they follow, the interpretable models they use, and the surface representation of explanations provided. The article concludes by identifying open questions and important challenges.}, keywords = {}, pubstate = {published}, tppubtype = {article} } Interpretability, explainability, and transparency are key issues to introducing artificial intelligence methods in many critical domains. This is important due to ethical concerns and trust issues strongly connected to reliability, robustness, auditability, and fairness, and has important consequences toward keeping the human in the loop in high levels of automation, especially in critical cases for decision making, where both (human and the machine) play important roles. Although the research community has given much attention to explainability of closed (or black) prediction boxes, there are tremendous needs for explainability of closed-box methods that support agents to act autonomously in the real world. Reinforcement learning methods, and especially their deep versions, are such closed-box methods. In this article, we aim to provide a review of state-of-the-art methods for explainable deep reinforcement learning methods, taking also into account the needs of human operators—that is, of those who make the actual and critical decisions in solving real-world problems. We provide a formal specification of the deep reinforcement learning explainability problems, and we identify the necessary components of a general explainable reinforcement learning framework. Based on these, we provide a comprehensive review of state-of-the-art methods, categorizing them into classes according to the paradigm they follow, the interpretable models they use, and the surface representation of explanations provided. The article concludes by identifying open questions and important challenges. |
2022 |
Vouros, George A Tutorial on Explainable Deep Reinforcement Learning: One framework, three paradigms and many challenges Inproceedings SETN 2022, ACM, 2022. @inproceedings{Vouros2022, title = {Tutorial on Explainable Deep Reinforcement Learning: One framework, three paradigms and many challenges}, author = {George A. Vouros}, doi = {10.1145/3549737.3549808}, year = {2022}, date = {2022-09-10}, booktitle = {SETN 2022}, publisher = {ACM}, abstract = {Interpretability, explainability and transparency are key issues to introducing Artificial Intelligence closed—box methods in many critical domains: This is important due to ethical concerns and trust issues strongly connected to reliability, robustness, auditability and fairness, and has important consequences towards keeping the human in the loop in high levels of automation, especially in critical cases for decision making. Reinforcement learning methods, and especially their deep versions, are closed-box methods that support agents to act autonomously in the real world. This tutorial will provide a formal specification of the deep reinforcement learning explainability problems, and will present the necessary components of a general explainable reinforcement learning framework. Based on this framework will provide distinct explainability paradigms towards solving explainability problems, with examples from state-of-the-art methods and real-world cases. The tutorial will conclude identifying open questions and important challenges. The tutorial is based on the survey paper on “Explainable Deep Reinforcement Learning” State of the Art and Challenges”}, keywords = {}, pubstate = {published}, tppubtype = {inproceedings} } Interpretability, explainability and transparency are key issues to introducing Artificial Intelligence closed—box methods in many critical domains: This is important due to ethical concerns and trust issues strongly connected to reliability, robustness, auditability and fairness, and has important consequences towards keeping the human in the loop in high levels of automation, especially in critical cases for decision making. Reinforcement learning methods, and especially their deep versions, are closed-box methods that support agents to act autonomously in the real world. This tutorial will provide a formal specification of the deep reinforcement learning explainability problems, and will present the necessary components of a general explainable reinforcement learning framework. Based on this framework will provide distinct explainability paradigms towards solving explainability problems, with examples from state-of-the-art methods and real-world cases. The tutorial will conclude identifying open questions and important challenges. The tutorial is based on the survey paper on “Explainable Deep Reinforcement Learning” State of the Art and Challenges" |
2020 |
Karampelas, Andreas; Vouros, George A Time and Space Efficient Large Scale Link Discovery using String Similarities Journal Article Fundamenta Informaticae, 172 , pp. 299-325, 2020, ISSN: 0169-2968 (P). @article{351, title = {Time and Space Efficient Large Scale Link Discovery using String Similarities}, author = {Andreas Karampelas and George A Vouros}, url = {https://content.iospress.com/articles/fundamenta-informaticae/fi1906?resultNumber=0&totalResults=158&start=0&q=Time+and+Space+Efficient&resultsPageSize=10&rows=10}, doi = {10.3233/FI-2020-1906}, issn = {0169-2968 (P)}, year = {2020}, date = {2020-02-01}, journal = {Fundamenta Informaticae}, volume = {172}, pages = {299-325}, keywords = {}, pubstate = {published}, tppubtype = {article} } |
Kotis, Konstantinos; Vouros, George A; Spiliotopoulos, Dimitris Ontology engineering methodologies for the evolution of living and reused ontologies: status, trends, findings and recommendations Journal Article The Knowledge Engineering Review, 35 , 2020. @article{358, title = {Ontology engineering methodologies for the evolution of living and reused ontologies: status, trends, findings and recommendations}, author = {Konstantinos Kotis and George A Vouros and Dimitris Spiliotopoulos}, url = {https://doi.org/10.1017/S0269888920000065}, doi = {https://doi.org/10.1017/S0269888920000065}, year = {2020}, date = {2020-01-01}, journal = {The Knowledge Engineering Review}, volume = {35}, keywords = {}, pubstate = {published}, tppubtype = {article} } |
Vouros, George A; Glenis, Apostolis; Doulkeridis, Christos The delta big data architecture for mobility analytics Conference 2020 IEEE Sixth International Conference on Big Data Computing Service and Applications (BigDataService), IEEE IEEE, Oxford, UK, 2020. @conference{359, title = {The delta big data architecture for mobility analytics}, author = {George A Vouros and Apostolis Glenis and Christos Doulkeridis}, year = {2020}, date = {2020-01-01}, booktitle = {2020 IEEE Sixth International Conference on Big Data Computing Service and Applications (BigDataService)}, publisher = {IEEE}, address = {Oxford, UK}, organization = {IEEE}, keywords = {}, pubstate = {published}, tppubtype = {conference} } |
2019 |
G.Vouros, ; Santipantakis, G; Doulkeridis, C; Vlachou, A; Andrienko, G; Andrienko, N; Fuchs, G; Martinez, Miguel Garcia; Cordero, Jose Manuel Garcia Journal Of Data Semantics, 8 , 2019. @article{352, title = {The datAcron Ontology for the Specification of Semantic Trajectories: Specification of Semantic Trajectories for Data Transformations Supporting Visual Analytics}, author = {G.Vouros and G Santipantakis and C Doulkeridis and A Vlachou and G Andrienko and N Andrienko and G Fuchs and Miguel Garcia Martinez and Jose Manuel Garcia Cordero}, url = {http://link.springer.com/article/10.1007/s13740-019-00108-0}, doi = {10.1007/s13740-019-00108-0}, year = {2019}, date = {2019-11-01}, journal = {Journal Of Data Semantics}, volume = {8}, chapter = {235}, keywords = {}, pubstate = {published}, tppubtype = {article} } |
Vouros, G; Vlachou, A; Doulkeridis, C; Glenis, A; Santipantakis, G Efficient Spatio-temporal RDF Query Processing in Large Dynamic Knowledge Bases Conference SAC 2019, 2019. @conference{346, title = {Efficient Spatio-temporal RDF Query Processing in Large Dynamic Knowledge Bases}, author = {G Vouros and A Vlachou and C Doulkeridis and A Glenis and G Santipantakis}, year = {2019}, date = {2019-01-01}, booktitle = {SAC 2019}, keywords = {}, pubstate = {published}, tppubtype = {conference} } |
Doulkeridis, Christos; Qu, Qiang; Vouros, George A; ~a, Jo Guest Editorial: Special issue on mobility analytics for spatio-temporal and social data. Journal Article GEOINFORMATICA, 23 , 2019. @article{353, title = {Guest Editorial: Special issue on mobility analytics for spatio-temporal and social data.}, author = {Christos Doulkeridis and Qiang Qu and George A Vouros and Jo ~a}, url = {https://link.springer.com/article/10.1007%2Fs10707-019-00374-x}, year = {2019}, date = {2019-01-01}, journal = {GEOINFORMATICA}, volume = {23}, chapter = {235}, keywords = {}, pubstate = {published}, tppubtype = {article} } |
2018 |
Santipantakis, G; Doulkeridis, C; Vouros, G; Vlachou, A MaskLink: Efficient Link Discovery for Spatial Relations via Masking Areas Journal Article arxiv, 2018. @article{336, title = {MaskLink: Efficient Link Discovery for Spatial Relations via Masking Areas}, author = {G Santipantakis and C Doulkeridis and G Vouros and A Vlachou}, url = {http://arxiv.org/abs/1803.01135}, year = {2018}, date = {2018-03-01}, journal = {arxiv}, keywords = {}, pubstate = {published}, tppubtype = {article} } |
Vouros, George A; et al., Big Data Analytics for Time Critical Mobility Forecasting: Recent Progress and Research Challenges Proceeding 2018. @proceedings{329, title = {Big Data Analytics for Time Critical Mobility Forecasting: Recent Progress and Research Challenges}, author = {George A Vouros and et al.}, year = {2018}, date = {2018-01-01}, journal = {EDBT 2018}, keywords = {}, pubstate = {published}, tppubtype = {proceedings} } |