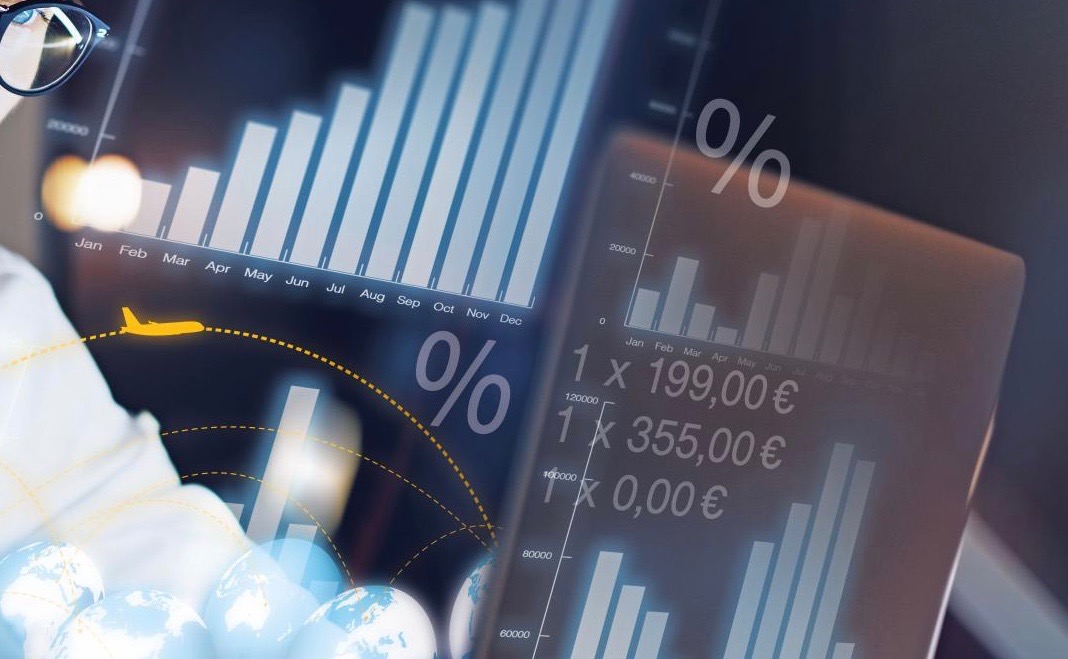
The overall goal of SIMBAD is to advance the state-of-the-art in ATM performance modelling through the combination of model-based approaches and data-driven methods, taking advantage of the opportunities opened by recent advances in big data technologies and data science.
The approach proposed by SIMBAD relies on three main pillars:
- the estimation of hidden variables in aircraft trajectory models,
- the development of new traffic pattern clustering and classification techniques, and
- the application of active learning meta-modelling to large-scale traffic simulations.
Duration
2020 – 2022
Objectives
The specific objectives of the project are the following:
1. Explore the use of machine learning techniques for the modelling of trajectories and estimation of hidden variables from historical air traffic data.
2. Develop new machine learning algorithms for traffic pattern classification.
3. Investigate the use of active learning metamodelling to enable a more efficient exploration of the input‑output space of complex ATM simulation models.
4. Demonstrate and evaluate the newly developed techniques in order to assess their maturity, derive recommendations on how to apply them to ATM performance assessment, and propose a roadmap for the transition of the project results to the next stages of the R&D cycle.
Contributions Expected
SIMBAD is expected to make the following contributions:
1. Better estimation of hidden variables: SIMBAD will explore the use of machine learning techniques in combination with state-of-the-art trajectory prediction and optimisation models to increase the available knowledge regarding hidden variables related to AU behaviour.
2. Improved data-driven trajectory prediction models: SIMBAD will advance the state-of-the-art in data-driven trajectory prediction models. The contributions in this area will be mainly two: (i) we will develop trajectory prediction approaches based on deep reinforcement learning that will extend existing single-agent approaches into multi-agent reinforcement learning methods able to take into account interactions and dependencies between different trajectories; (ii) we will explore how to use the reinforcement learning imitation process for the identification of AUs’ preferences and behaviour in relation to ATFCM measures.
3. Comprehensive multi-scale classification of traffic patterns in the ECAC area: as opposed to the US, research on traffic pattern classification is very limited in Europe. SIMBAD will contribute to filling this gap by advancing the state-of-the-art in two ways: (i) we will develop a multiscale approach, able to identify traffic patterns at different spatial and temporal scales; (ii) we will apply the newly developed methods to ECAC area traffic, identifying not only relevant traffic patterns under normal operations, but also different categories of “disrupted operations”.
4. Introduction to active learning metamodelling techniques in ATM: to best of our knowledge, the application of active learning metamodelling techniques to large-scale traffic microsimulation models has not yet been explored in ATM.
Partners
Nommon , Spain
CRIDA, Spain
UPC, Spain
UPRC, Greece
Fraunhofer (IAIS), Germany
Eurocontrol, EU
Leave a Reply
You must be logged in to post a comment.