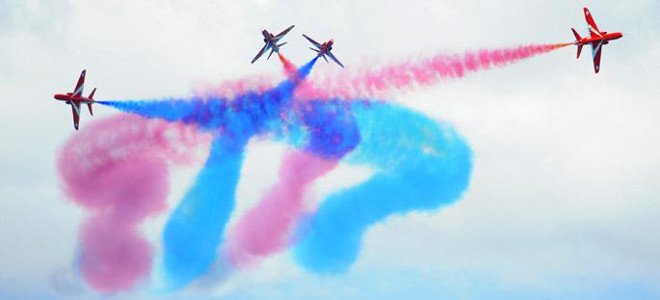
While data-driven methods aim to build models for trajectory planning and conflicts resolution, incorporating stakeholders’ interests and preferences, the multi-agent reinforcement learning (MARL) approach aims to address complexity phenomena due to traffic and resolve conflicts between multiple trajectories, simultaneously. Towards that goal we aim to formulate the problem as a Markov Decision Making Process, i.e. MDP, and apply multi-agent reinforcement learning (MARL) methods to resolve it.
Duration
2019 – 2020
Objective
The objective of this PhD is to explore and present novel algorithms towards planning conflict-free trajectories at the pre-tactical phase of operations in computationally efficient ways, for large number of trajectories in airspaces comprising multiple FIRs, following a methodology combining data-driven and agent-based approaches
Challenges
- Addressing conflict-free trajectory planning among multiple flights, simultaneously, while considering complex ATM phenomena and operational constraints.
- Addressing optimization in trajectory planning taking into account multiple objectives concerning airspace use, cost indicators, preferences and constraints of stakeholders involved.
- Follow a data-driven approach to reveal stakeholders’ preferences on trajectories followed and on resolving conflicts.
- Addressing complex air traffic management phenomena due to traffic.
- Addressing scalability: Coordinating between different FIRs for large number of flights.
Contributions expected
- Contribute towards collaborative decision making for conflict-free trajectory planning involving multiple stakeholders (Airspace users, ANSPs, Network Manager, Traffic Controllers).
- Reveal preferences and constraints concerning trajectories and airspace use using data-driven approaches.
- Explore and provide an understanding of how resolution of conflicts among trajectories is affected by preferences and constraints concerning trajectories and airspace use.
- Reduce Air Traffic Controllers workload due to conflicts at the tactical stage, while enabling performing effective conflict-free planning at the planning stage of operations.
- Explore and develop where appropriate spatiotemporal abstractions in MARL techniques to address large-scale complex phenomena in real-world settings.
- Contributions to the ATM Master Plan:
- Improved operations productivity via contributions to improved collaborative planning tools accounting for complex phenomena due to traffic.
- Increasing predictability via efficient operation plans devised at the pre-tactical stage, reducing buffers and uncertainty.
- Minimizing the impact of delays due to ATM factors.
- Reduce number of flight inefficiencies due to tactical ATC actions, supporting better planning of operations for Airspace Users.
Leave a Reply
You must be logged in to post a comment.